Unlock the secrets behind artificial intelligence (AI) and quantum computing, two revolutionary technologies that are shaping our future. While AI has made remarkable strides in recent years, enabling machines to learn, reason, and make decisions like never before, quantum computing promises to supercharge these capabilities by harnessing the mind-bending properties of quantum mechanics. Quantum computing could potentially solve complex problems that are intractable for classical computers, opening up new frontiers in AI. However, the intersection between these two cutting-edge fields is still in its early stages. Join us as we explore the current state of quantum AI, the challenges that lie ahead, and the incredible possibilities that await us in this quantum-powered future.
The Basics of Quantum Computing
Qubits: The Building Blocks
At the heart of quantum computing lie qubits, or quantum bits. Unlike classical bits, which can only be in one of two states (0 or 1), qubits can exist in a superposition of multiple states simultaneously. This property allows quantum computers to perform certain calculations exponentially faster than traditional computers.
Qubits are typically made from subatomic particles such as electrons or photons, which can be manipulated using lasers or microwave beams. When multiple qubits are entangled, they form a powerful system capable of parallel processing. As Dr. Sarah Johnson, a quantum physicist at MIT, explains, “Entanglement is like having a group of dancers who can coordinate their movements instantly, no matter how far apart they are.”
However, qubits are also incredibly fragile and susceptible to interference from the environment. Maintaining their stability requires advanced error correction techniques and ultra-cold temperatures near absolute zero. Despite these challenges, researchers are making steady progress in building larger and more reliable quantum systems.
As quantum computers become more powerful, they hold the potential to revolutionize fields such as cryptography, drug discovery, and machine learning. While still in its early stages, the intersection of quantum computing and AI promises to unlock new frontiers in science and technology.
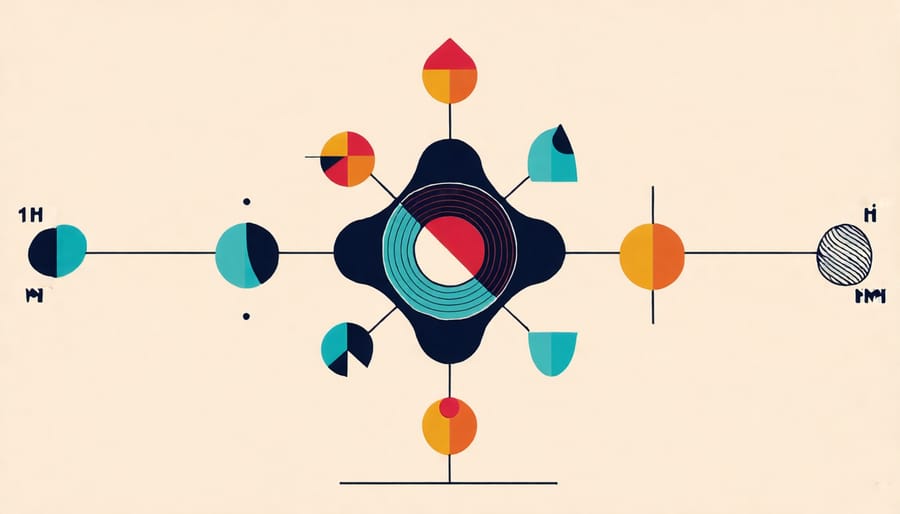
Superposition and Entanglement
Superposition and entanglement are two fundamental principles of quantum mechanics that enable quantum computing’s vast potential. In superposition, a quantum bit, or qubit, can exist in multiple states simultaneously, unlike classical bits limited to binary 0 or 1 states. This allows quantum computers to perform numerous calculations in parallel, exponentially increasing computing power as more qubits are added.
Entanglement occurs when two or more qubits become correlated, such that measuring one instantly affects the others, regardless of distance. This “spooky action at a distance,” as Einstein famously described it, is a key feature of the Copenhagen Interpretation of quantum mechanics. Entanglement allows quantum computers to process vast amounts of data and tackle complex problems that are intractable for classical computers.
While quantum superposition and entanglement offer immense computing potential, harnessing them remains an engineering challenge. Qubits are highly sensitive to external interference and must be maintained in extreme conditions, such as near absolute zero temperatures. Despite these hurdles, researchers continue to make strides in developing stable, scalable quantum systems. As quantum computing advances, it holds promise for revolutionizing fields like cryptography, drug discovery, and artificial intelligence, pushing the boundaries of what’s possible with computation.
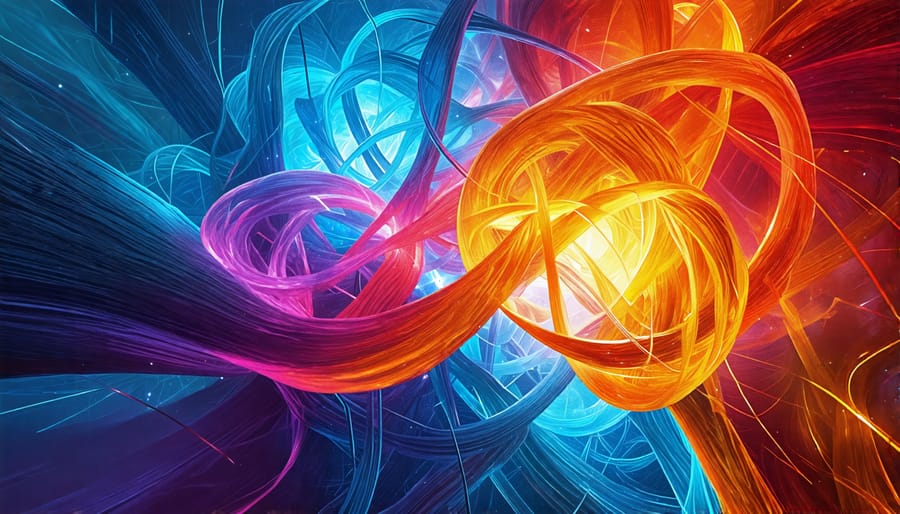
AI’s Current Landscape
The field of artificial intelligence (AI) has made remarkable strides in recent years, revolutionizing industries and transforming the way we live and work. From virtual assistants and autonomous vehicles to personalized recommendations and predictive analytics, AI has become an integral part of our daily lives. However, despite its impressive capabilities, the current landscape of AI primarily relies on classical computing systems.
Classical computing, which has been the foundation of computing for decades, operates on binary principles, using bits that can be either 0 or 1. This approach has proven to be highly effective for many tasks, enabling AI systems to process vast amounts of data, recognize patterns, and make decisions based on complex algorithms. However, as AI continues to evolve and tackle more challenging problems, the limitations of classical computing are becoming increasingly apparent.
One of the primary limitations of classical computing in AI is its scalability. As the complexity of AI models grows, so does the computational power required to train and run them. This can lead to long training times, high energy consumption, and significant costs. Additionally, certain problems, such as optimization and simulation of complex systems, can be intractable for classical computers due to the exponential growth of computational complexity.
Another challenge facing AI in the classical computing paradigm is the handling of uncertainty and ambiguity. Many real-world problems involve incomplete or noisy data, which can be difficult for deterministic algorithms to navigate. Classical computing struggles to efficiently represent and manipulate the probabilistic nature of such problems, limiting the effectiveness of AI in these domains.
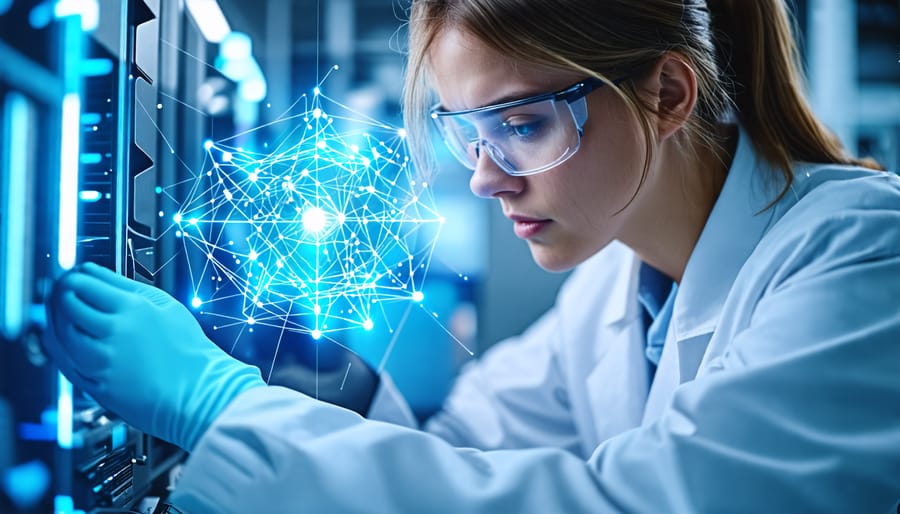
The Quantum-AI Intersection
Quantum Machine Learning
Quantum machine learning is an emerging field that combines the principles of quantum computing with machine learning algorithms. By leveraging the unique properties of quantum systems, such as superposition and entanglement, quantum machine learning has the potential to revolutionize AI and solve complex problems that are intractable for classical computers.
One of the most promising applications of quantum machine learning is in the area of optimization. Many machine learning tasks, such as training neural networks or finding the best solution to a problem, involve searching through a vast space of possibilities. Quantum algorithms, like the quantum approximate optimization algorithm (QAOA), can potentially find optimal solutions much faster than classical algorithms.
Another exciting application is in the field of quantum-enhanced feature spaces. By mapping classical data into a quantum state, researchers can exploit the exponentially large quantum feature space to uncover hidden patterns and correlations that are difficult to detect using classical methods. This could lead to more accurate and efficient machine learning models for tasks like image recognition, natural language processing, and drug discovery.
However, the field of quantum machine learning is still in its early stages, and there are significant challenges to overcome. One major hurdle is the limited size and noise of current quantum hardware, which restricts the complexity of quantum machine learning algorithms that can be implemented. Additionally, there is a lack of standard datasets and benchmarks to evaluate the performance of quantum machine learning models.
Despite these challenges, researchers are making rapid progress in developing new quantum machine learning algorithms and improving the capabilities of quantum hardware. As the field advances, quantum machine learning has the potential to unlock unprecedented insights and capabilities in AI, pushing the boundaries of what is possible with computational intelligence.
Research and Real-World Examples
While the intersection of AI and quantum computing is still in its early stages, several notable research projects and real-world applications have emerged. In 2020, Google’s quantum computer achieved “quantum supremacy” by performing a task in 200 seconds that would take a classical supercomputer 10,000 years. This milestone demonstrated the potential of quantum computing to revolutionize AI and machine learning.
IBM has also made significant strides in quantum AI research. Their quantum computer, IBM Q, has been used to develop quantum algorithms for machine learning tasks such as classification and clustering. These algorithms leverage the unique properties of quantum systems to potentially outperform classical AI approaches.
Another exciting application is in the field of renewable energy. Researchers are exploring how quantum computing can optimize AI-powered renewable energy systems, such as wind and solar farms. By leveraging quantum optimization algorithms, AI could more efficiently manage and distribute renewable energy resources, leading to a greener future.
Moreover, quantum AI has the potential to accelerate drug discovery and development. Quantum computers can simulate complex molecular systems, enabling AI to identify promising drug candidates more quickly and accurately than traditional methods. This could lead to breakthrough treatments for diseases like cancer and Alzheimer’s.
As research continues to progress, the synergy between AI and quantum computing holds immense promise. From tackling climate change to advancing healthcare, the real-world applications of quantum AI are poised to reshape our world in profound ways.
Future Outlook
The future of quantum AI holds immense promise, with the potential to revolutionize various fields and tackle some of humanity’s most complex challenges. As quantum computing technology continues to advance, researchers are optimistic about the development of more sophisticated quantum AI systems capable of processing vast amounts of data and performing intricate computations far beyond the reach of classical computers.
One of the most exciting prospects is the application of quantum AI in drug discovery and healthcare. By leveraging the power of quantum algorithms, AI systems could rapidly analyze massive molecular databases, leading to the identification of novel drug candidates and personalized treatment strategies. This could accelerate the development of life-saving medications and transform the way we approach medical research.
Another area where quantum AI shows great potential is in the realm of optimization and logistics. From traffic management and supply chain optimization to financial portfolio analysis, quantum AI algorithms could help find optimal solutions to complex problems in a fraction of the time required by classical methods. This could lead to significant improvements in efficiency, cost savings, and decision-making across various industries.
However, the path to realizing the full potential of quantum AI is not without challenges. One of the primary hurdles is the development of reliable and scalable quantum hardware. Current quantum computers are still prone to errors and have limited qubit counts, which restricts the complexity of the algorithms they can run. Researchers are working tirelessly to improve the stability and coherence of quantum systems, but there is still a long way to go before we can harness the true power of quantum computing.
Another challenge lies in the development of quantum algorithms and software that can effectively utilize the unique properties of quantum systems. This requires a deep understanding of both quantum mechanics and classical AI techniques, as well as the ability to translate complex problems into quantum circuits. As Professor John Preskill of Caltech notes, “We need to build a bridge between the quantum world and the classical world of AI.”
Despite these challenges, the scientific community remains optimistic about the future of quantum AI. With continued research and collaboration across disciplines, we can expect to see significant advancements in the coming years. As quantum computing and AI continue to evolve and converge, the possibilities for groundbreaking discoveries and transformative applications are truly limitless. The future of quantum AI is not just about pushing the boundaries of computation; it’s about unlocking new frontiers of human knowledge and shaping a better world for generations to come.