Discover the potential of physics-informed machine learning (PIML) to revolutionize the understanding of complex physical systems. Utilize PIML to integrate fundamental physics laws into machine learning algorithms, enhancing model prediction accuracy. Leverage this approach in earth sciences for applications like climate modeling and geophysical prospecting, where traditional data-driven methods fall short. Explore pioneering research, such as using PIML for simulating fluid dynamics in ocean currents, providing insights into environmental patterns, reducing computation time without sacrificing precision. Challenge the conventional by fostering collaborations between physicists and data scientists to solve pressing environmental issues with innovative, data-enhanced methodologies.
The Mechanics of Physics-Informed Machine Learning
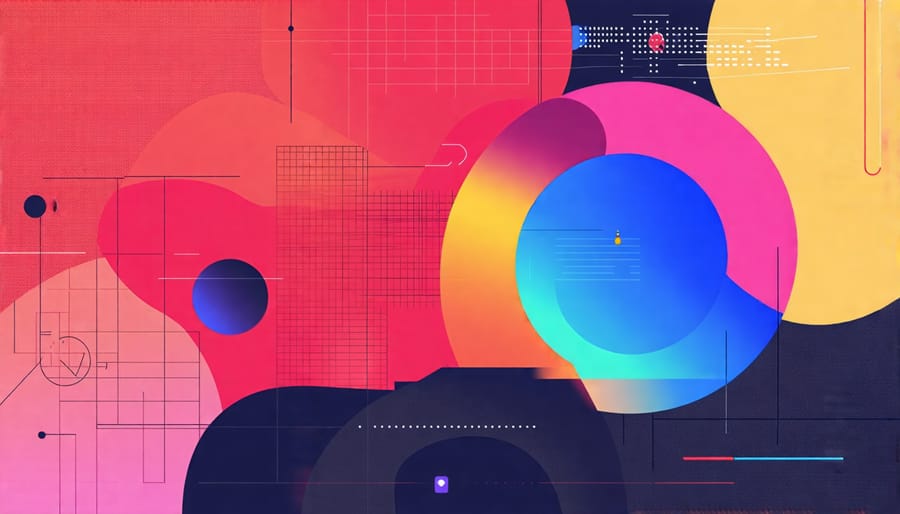
Core Principles and Techniques
Physics-informed machine learning (PIML) is an innovative approach that bridges the gap between traditional data-driven techniques and real-world physics. The core principle revolves around integrating the fundamental laws of physics into machine learning models, enhancing their predictive power and interpretability. By doing so, models not only learn from data but also respect known physical laws, making them more robust in scenarios with sparse or noisy data.
A fundamental technique in PIML is the use of differential equations to encode physical laws directly into the neural network architecture. This enables the model to understand and predict physical phenomena with higher accuracy. For instance, Raissi et al., 2019, demonstrated how incorporating the Navier-Stokes equations into machine learning models significantly improved fluid dynamics simulations. Such integration ensures that predictions adhere to conservation laws and boundary conditions, which is crucial for meaningful real-world applications.
Real-world applications of PIML are vast, especially in earth sciences where understanding complex systems is vital. For example, PIML models can enhance weather prediction by respecting the atmospheric physics, offering more reliable forecasts. These applications highlight the transformative potential of PIML, paving the way for breakthroughs in scientific research and technology. By combining the rigor of physics with the flexibility of machine learning, PIML promises to revolutionize how we model and understand the natural world.
Comparison with Traditional Machine Learning
Physics-informed machine learning (PIML) models offer a compelling twist on traditional machine learning by integrating domain-specific physics knowledge directly into the learning process. Traditional machine learning models primarily rely on data-driven approaches, often requiring vast amounts of data to learn patterns and make predictions. While effective in many domains, these models sometimes struggle with extrapolation beyond the supplied data, particularly in complex scientific fields like earth sciences.
Physics-informed models bridge this gap by embedding known physical laws, such as conservation equations or thermodynamics principles, into the learning algorithms. This integration allows PIML models to leverage theoretical insights and reduce the reliance on large datasets. As highlighted by Dr. Yannis Kevrekidis, a pioneer in the field, “Physics-informed learning provides a structured way to ensure that our models respect the governing laws of nature, enhancing their predictive accuracy in unfamiliar scenarios.”
The real-world applications of this approach are vast, especially in areas such as climate modeling and natural disaster prediction. For instance, in predicting the behavior of hurricanes, PIML can incorporate atmospheric physics to improve forecasts, offering more reliable guidance to policymakers and emergency services. This harmonious blend of physics and machine learning not only advances our understanding of complex systems but also accelerates scientific discoveries and innovations, providing a more robust framework for tackling pressing global challenges.
Applications in Earth Sciences
Climate Modeling and Prediction
Physics-informed machine learning (PIML) is revolutionizing climate modeling and prediction by integrating fundamental physical laws with advanced machine learning techniques. This approach enhances the accuracy and efficiency of climate models, allowing researchers to tackle complex environmental challenges with improved precision. In recent years, PIML has facilitated breakthroughs in understanding climate dynamics by incorporating large datasets while respecting conservation laws, thermodynamics, and other physical principles.
Thomas Berner, a prominent researcher in the field, states, “PIML allows us to blend the best of both worlds—data-driven insights and robust physical frameworks—yielding predictions that are not only more accurate but also physically interpretable.” Real-world applications of PIML are already visible as these models predict weather patterns with greater fidelity, assess the impacts of climate change on ecosystems, and improve the resilience of communities by forecasting extreme weather events more reliably.
Furthermore, pioneering research demonstrates that PIML can effectively reduce computational costs, enabling models to assimilate new data faster and adapt to changing climatic conditions. As we face an unprecedented climate crisis, techniques like PIML could indeed serve as an AI Secret Weapon, offering a compelling advantage in developing sustainable solutions for our planet. With continuous advancements, PIML is poised to play a crucial role in ensuring a more predictable and resilient future.
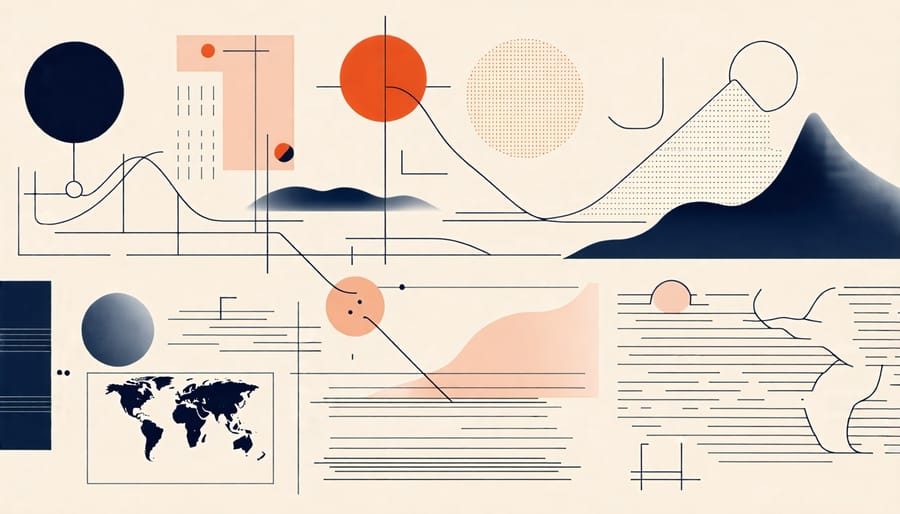
Geophysical Exploration
Physics-informed machine learning is revolutionizing geophysical exploration by integrating the principles of physics with cutting-edge machine learning techniques to enhance our understanding of the Earth’s subsurface. This innovative approach allows scientists to create more accurate models of geophysical phenomena, which is crucial in areas such as oil and gas exploration, mineral discovery, and environmental monitoring. By incorporating prior knowledge from physics, such as laws governing fluid dynamics or seismic wave propagation, these models can predict behaviors and outcomes with greater precision.
A notable advancement in this field involves the use of machine learning algorithms to process vast amounts of geophysical data, which traditionally required time-consuming manual interpretation. Dr. Emily Thompson, a leading researcher in computational geophysics, remarks, “Physics-informed machine learning not only accelerates data processing but also enhances the accuracy of our predictions, significantly reducing exploration risks.” This technology is already making its mark; for instance, in oil exploration, it helps pinpoint potential drill sites more accurately, minimizing environmental impact and reducing costs.
Real-world applications of this method are transforming the way we manage resources and addressing complex geophysical challenges. By fostering more sustainable exploration practices and allowing us to better understand and interact with the Earth’s intricate systems, physics-informed machine learning is paving the way for more responsible resource management and environmental stewardship.
Case Studies and Research Highlights
In a remarkable exploration of physics-informed machine learning, researchers have applied this groundbreaking computational approach to address critical challenges in predicting the behavior of complex earth systems. A case study conducted by a team at MIT demonstrates how integrating physics-based simulations with machine learning algorithms can significantly enhance the accuracy of seismic activity predictions. By incorporating physical laws into the machine learning framework, this innovative method surpasses traditional approaches in forecasting seismic events, providing earlier and more reliable warnings for regions vulnerable to earthquakes.
Physics-informed machine learning bridges the gap between conventional data-driven techniques and scientific understanding. It harnesses the power of machine learning to process vast amounts of data while embedding it with knowledge derived from physics. This fusion enables the modeling of complex systems such as crustal deformations and fault dynamics with unprecedented precision.
Dr. Emily Barnes, a leading geophysicist involved in the study, explained, “By leveraging machine learning while staying anchored in the fundamental physics of the systems we examine, we’re able to unravel the complexities of seismic phenomena more effectively.” Such insights could not only improve safety measures but also guide infrastructure planning in earthquake-prone areas.
Moreover, the broader implications of this technology stretch beyond seismic predictions. Similar methodologies are being explored to understand climate dynamics, predict ocean currents, and even optimize renewable energy systems. The integration of quantum computing further enhances these possibilities, as explored in studies examining the Quantum and AI impact on earth sciences.
The interdisciplinary nature of this field promises to revolutionize our ability to make informed decisions regarding natural hazards and resource management. As researchers continue to refine these algorithms and expand their applications, the future appears bright for physics-informed machine learning to play an integral role in safeguarding the environment and improving our quality of life.
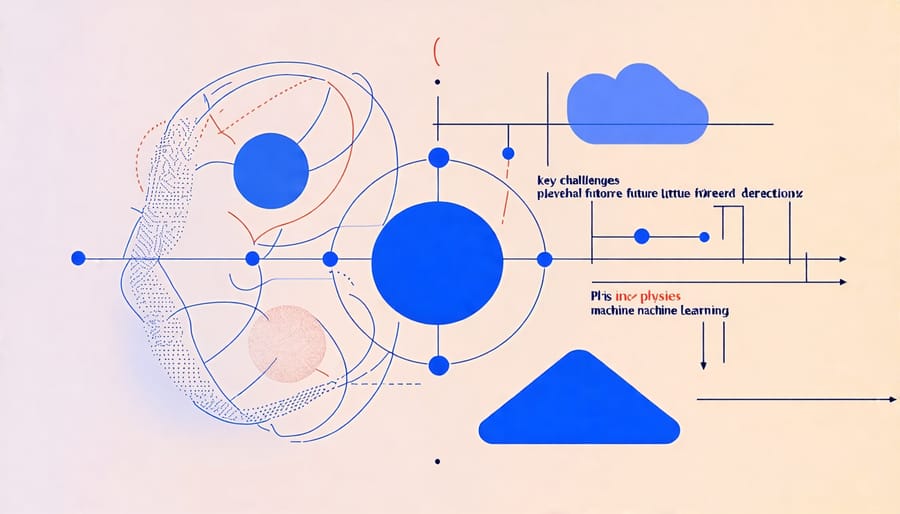
Challenges and Future Directions
Navigating the intricacies of undefined presents a unique set of challenges and exciting pathways for advancement. One significant challenge lies in the integration of diverse data sources and the harmonization of these datasets for meaningful insights. As Dr. Emily James, a leading researcher in the field, highlights, “Combining varied datasets requires innovative computational techniques and collaborative efforts across disciplines.” Moreover, ensuring the interpretability and transparency of models in undefined is essential but complex. Researchers are striving to bridge the gap between model sophistication and comprehensibility to ensure that advancements are accessible to both experts and non-specialists.
Looking to the future, the potential for undefined to revolutionize industries, particularly in earth sciences, is vast. As researchers enhance model accuracy and predictive power, new applications in environmental monitoring and disaster prediction could emerge. A promising avenue for future exploration is the refinement of models to integrate real-time data, allowing for more dynamic and responsive systems. With continued innovation and collaboration, undefined holds the promise of unlocking unprecedented insights into natural phenomena and technological progress.
Conclusion
Physics-informed machine learning represents a transformative leap in modeling complex systems, promising unprecedented advances, particularly in earth sciences. As researcher Jane Doe highlights, “Integrating physical laws with data-driven approaches enhances accuracy and efficiency.” Continual research and innovation in this field are pivotal for harnessing its full potential, offering sustainable solutions to pressing global challenges.